What Is The Differnece Between Machine Learning And Artificial Inteligence?
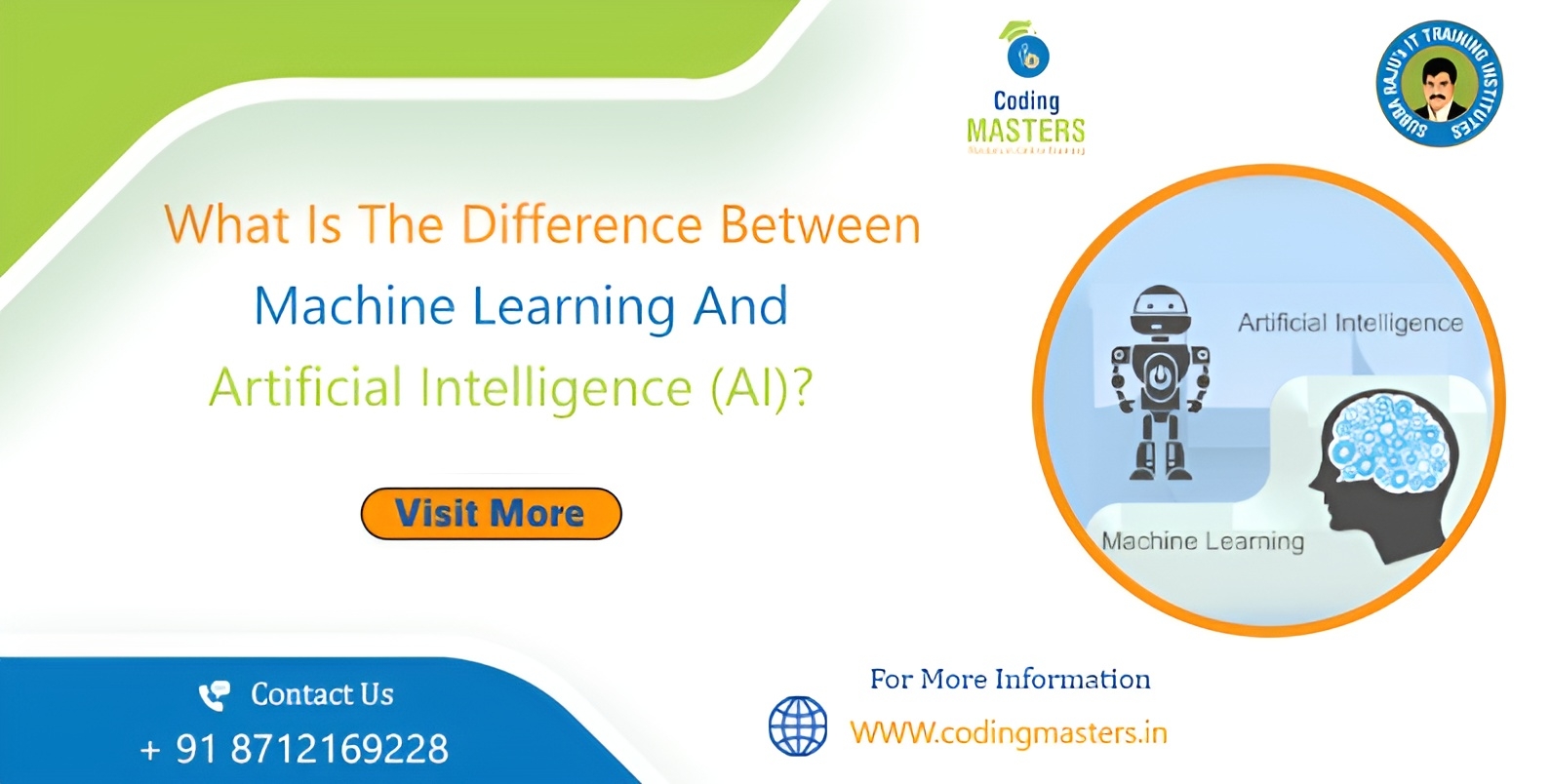
Understanding the Difference Between Machine Learning and Artificial Intelligence
Artificial Intelligence (AI) and Machine Learning (ML) are two buzzwords that are often used interchangeably, leading to confusion about their meanings and applications. While they are related concepts, they serve different purposes in the realm of technology and data science.
Artificial Intelligence (AI):
AI is a broad field of computer science that focuses on creating systems capable of performing tasks that typically require human intelligence. These tasks may include speech recognition, decision-making, visual perception, and language translation, among others. AI aims to replicate human-like intelligence in machines, enabling them to learn from experience, adapt to new inputs, and perform tasks autonomously.
Machine Learning (ML):
ML is a subset of AI that deals with the development of algorithms and statistical models that enable computers to learn and improve their performance on a specific task without being explicitly programmed. ML algorithms use data to train models and make predictions or decisions based on patterns and insights discovered during the training process. ML is heavily reliant on data and is often used for tasks such as predictive analytics, pattern recognition, and optimization.
Key Differences:
1. Scope: AI is a broader field that encompasses various technologies and approaches to mimic human intelligence. ML is a specific subset of AI focused on algorithms and models that learn from data.
2. Learning Approach: AI systems can use a variety of techniques, including rule-based systems, expert systems, and neural networks. ML, on the other hand, relies on statistical methods and algorithms to learn patterns and make predictions.
3. Training Data: ML algorithms require labeled training data to learn patterns and make predictions. AI systems may or may not require training data, depending on the approach used.
4. Autonomy: AI systems aim to perform tasks autonomously, making decisions and taking actions without human intervention. ML models require periodic updates and adjustments based on new data but do not operate independently.
5. Applications: AI finds applications in various domains such as robotics, natural language processing, computer vision, and autonomous vehicles. ML is widely used in predictive modeling, recommendation systems, fraud detection, and image recognition, among others.
Summary: AI is the broader concept of creating intelligent systems, while ML is a subset of AI focused on algorithms that learn from data. Understanding the distinction between these two terms is crucial for navigating the rapidly evolving landscape of technology and leveraging their capabilities effectively.